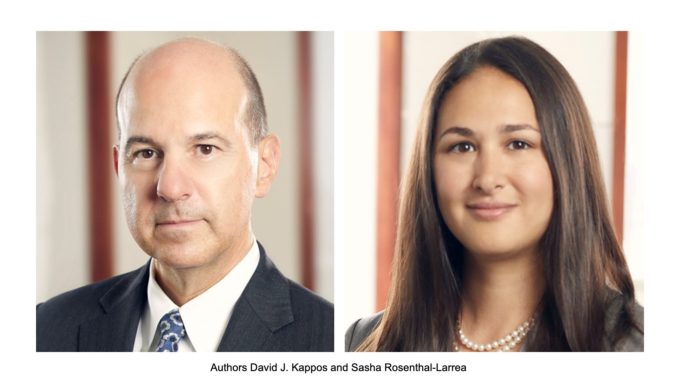
ChatGPT and Text Fakes—Sensible Policy to Balance Growth and Risk
By David J. Kappos, Sasha Rosenthal-Larrea, Daniel M. Barabander, and Leslie Liu - Edited by Edwin Farley and Pablo A. Lozano
Introduction
ChatGPT, a text generative model from OpenAI, has Google worried. OpenAI’s GPT-4 can now, for instance, write poems as well as research papers. While generative AI text models have many benign or beneficial use cases, others are more ominous. Generative text models can explain quantum physics, plan your travel itinerary, and even opine on ethics. But they can also be a powerful tool for those wishing to spread misinformation. The thin line between the useful, productive purposes and the destructive, nefarious purposes is unique to this technology.
It is impossible to have a conversation about the dark side of AI-generated content without discussing deepfakes. Coined in 2017 by an eponymous Reddit user, the term “deepfakes” commonly refers to synthesized images or videos. However, deepfakes are part of a larger category of “synthetic media,” which is “any media which has been created or modified through the use of artificial intelligence.” Notable uses of this technology include text, audio, and video fakes.
In China, synthetic media is becoming increasingly widespread. Deepfake videos and images have mostly been used recreationally through apps such as Zao and Avatarify. Generative AI text is also gaining momentum, with WuDao 2.0, a natural language processing model, taking over headlines in 2021. On March 16, 2023, Chinese internet search giant Baidu unveiled a new generative chatbot, Ernie Bot.
On January 10, 2023, the Cyberspace Administration of China (CAC) began to administer a new law that regulates AI systems used to produce synthetic media, what the law calls “deep synthesis technology.” The “Provisions on the Administration of Deep Synthesis Internet Information Services” (referred to as the “Deep Synthesis Law”) regulates how deep synthesis service providers in China—including providers of text, audio and video services—deliver applicable technology online. Commentators view the Deep Synthesis Law as being the first of its kind globally.
This article seeks to clarify the law and highlight considerations for U.S. policymakers. Commentaries surrounding the Deep Synthesis Law’s application to deep synthesis technology have focused on synthesized audio and video content. This myopia is at best incomplete and at worst misleading. Part I summarizes the Deep Synthesis Law. Part II discusses the current state of U.S. deep synthesis regulation. Finally, Part III argues that Congress should include “text fakes” on its regulatory radar and treat each type of deep synthesis technology in a way that reflects the potential harm it presents.
Part I: Deep Synthesis Law: Features and Misreads
There are three features of China’s Deep Synthesis Law that bear highlighting.
First, its requirements are built upon principles of labeling and, in some situations, consent. The law mandates labeling practices, which are akin to watermarking, for uses of deep synthesis technology “that may cause confusion or misidentification among the public.” This requires deep synthesis service providers to include a “conspicuous mark” in a “reasonable position” on the synthesized material, alerting users that the material is created using deep synthesis technology. Article 14 specifies that providers who use biometric information—which includes audio and visual fakes, but not text fakes—need to obtain individual consent from users.
Second, the law operationalizes its regulation by putting the burden of compliance solely on deep synthesis service providers, holding providers exclusively liable for noncompliance. Under Article 23, “deep synthesis service providers” is an umbrella term that includes all organizations and individuals who provide deep synthesis services. Under Article 22, service providers will be subject to both civil and criminal liabilities for violations. At the same time, service providers have significant leeway in determining how to implement the regulations, with Article 5 encouraging providers to self-police, subject to oversight by internet regulators.
Third, the Deep Synthesis Law represents the first instance of a national AI regulation that explicitly considers generative text. Previously, China passed Regulations on the Administration of Online Audio and Video Information Services, which went into effect on January 1, 2020, regulating only audio and media providers that use deep synthesis technology. The Deep Synthesis Law, on the other hand, defines “deep synthesis” to include, beyond audio and video, uses of text, such as “text generation, text style changes [and] conversation generation technology.” In a press release, regulators noted that the law’s explicit consideration of text and its ancillaries demonstrates their intent to combat fake news and disinformation.
The flood of attention on China’s Deep Synthesis Law has largely ignored the law’s explicit inclusion of text fakes as part of deep synthesis technology. For instance, CNBC noted the law as regulating “deepfakes,” which it defined as “synthetically generated or altered images or videos.” Reuters characterized the law as applying to “content providers that alter facial and voice data,” without mentioning text fakes. According to the Global Times, the regulations only require “synthetic videos and photos made using deep synthesis technology” to be clearly labeled. Other media outlets, such as AI News, simply reported that the CAC “announced rules to clampdown on deepfakes,” without elaborating on the type of technology regulated.
Part II: Current U.S. Regulations
The Wall Street Journal points out that the Deep Synthesis Law marks “one of the world’s first large-scale efforts to try to address one of the biggest challenges confronting society”—AI. As the first-mover in this regulatory space, China’s approach in its new law described above in Part I could set a standard for regulation of synthetic media technology in other jurisdictions. In contrast to the proactive Chinese regulators, U.S. regulators seem less concerned, at least as to domestic usage of deep synthesis technology. While China's law is certainly not the only possible regulatory avenue, the fact that Chinese regulators have promulgated such a law, unlike U.S. regulators, means they are aware and concerned of the dangers of text fakes. Individual U.S. states have enacted laws addressing visual deepfakes, but there is no coordinated or concrete action—especially regarding text fakes—at the federal level. While two laws pertinent to deepfakes have been introduced in Congress, the National Defense Authorization Act for Fiscal year 2020 (“NDAA”) and the DEEPFAKES Accountability Act, the former is merely research-based and the latter has yet to be passed.
On December 20, 2019, President Trump signed the NDAA into law, which took a research-based approach to deepfakes. The portion covering deepfakes is broken out into two parts. First, it requires the Director of National Intelligence to submit an annual report on deepfake capabilities—defined as the use of “machine-manipulated media and machine-generated text”—of foreign governments. Second, it establishes a competition to encourage research or commercialization of deepfake-detection technologies. Importantly, the NDAA only mandates a fact-gathering exercise, with no operative provision regulating conduct.
On the other hand, the DEEPFAKES Accountability Act has not advanced beyond the proposal stage in Congress. After it was introduced in the House in April 2021, it has been referred to various subcommittees, but without seeing further development. The Act includes both a “digital watermark” requirement and a “disclosure” requirement. The watermark requirement reads: “[a]ny advanced technological false personation record which contains a moving visual element shall contain an embedded digital watermark clearly identifying such record as containing altered audio or visual elements.” The disclosure requirement includes both a statement that identifies the material as having altered elements and a description of the extent of such alteration.
While similar in regulatory approach to China’s Deep Synthesis Law, the DEEPFAKES Act does not consider text fakes, nor does it make clear who is responsible for compliance, as the Chinese law does. The Act neither recognizes the danger of generative text nor specifies the party to be regulated. In defining “deepfake” as “any video recording, motion-picture film, sound recording, electronic image, or photograph, or any technological representation of speech or conduct substantially derivative thereof,” the Act excludes generative text from its purview. Further, while the Act provides criminal and civil penalties, it does not identify on whom the burden of compliance falls. As the next Part argues, future regulation of text fakes could build upon this law by including generative text and specifying responsible parties.
Part III: Future of U.S. Regulation
The Deep Synthesis Law provides three key insights for U.S. regulators. First, regulators should consider how text fakes could be misused to launch large-scale disinformation campaigns. Second, regulators should tailor laws to address the unique risks stemming from different uses of deepfake technology. Third, there should be a thoughtful debate around who should be responsible for implementing the regulations.
A. Dangers of Text Fakes
Text fakes encompass a perfect storm of features that lend themselves to large-scale disinformation campaigns. First, they are cheap and fast. Second, the technology has been shown to accentuate extremist viewpoints. Third, the public is not adequately informed of the existence or dangers of text fakes. These features could enable a foreign or domestic nefarious actor to use text fakes to do damage at a national level. A threat of this magnitude warrants a coordinated federal response, not merely a state-level patchwork.
Text fakes can spread misinformation like wildfire, culminating in a disinformation campaign. While misinformation is false information that is spread regardless of the intent to mislead, disinformation is misinformation that is spread intentionally. Text fakes’ capacity to lower the cost of disinformation campaigns while at the same time dramatically increasing their speed is particularly concerning. Moreover, when AI models enter the picture and work in conjunction with human operators, teams can produce disinformation at a frightening scale and speed, indiscriminately affecting people across state lines. Ahead of the 2016 U.S. election, Russian organizations employed hundreds of individuals to create fictitious social media accounts, with a goal of “spread[ing] distrust towards the candidates and the political system in general.” But this was a manual operation, expensive and time-consuming—it is not difficult to imagine how AI-generated text could have made Russia’s efforts all the more disruptive and far-reaching.
Text fake systems have displayed a proclivity towards adopting extremist positions, rendering them an inherently potent tool in disinformation campaigns. Research from Georgetown University’s Center for Security and Emerging Technology shows that “in several instances, GPT-3 spun its outputs to stances more extreme than those represented by the real articles . . . explicitly chose[n] to represent the extreme poles of ‘legitimate’ debate surrounding each topic.” In another test, GPT-3 eagerly portrayed President Trump as a “noble victim” of the January 6th Capitol Riot. Extremist positions are particularly effective at influencing public thought, making the technology’s tendency to adopt these views highly dangerous.
Compounding these unique risks of text fakes is an unaware public. Though the public has developed a healthy skepticism of synthesized images, it has not yet adjusted to an environment rife with synthesized text. Awareness about the technology is key for an individual to discern truth and fiction. On February 16, 2023, a recreational user of ChatGPT in Hangzhou, China, made headlines by creating a fake announcement that appeared to come from the city on changes in traffic patterns using the tool. The user sent the article into a WeChat group, where others mistakenly believed it was a legitimate and official announcement. The article spread rapidly, causing mayhem before it was taken down. This user had no ulterior motive, initially intending to simply “experiment with” ChatGPT’s capabilities. Part of the driver for the “success” of this “experiment” was the public’s unfamiliarity with the technology that made it possible—and how it was unprepared to doubt the text’s authenticity.
B. Tailoring Laws for Text Fakes
With the exception of the consent requirement from users whose biometric information is synthesized, China’s Deep Synthesis Law fails to tailor regulatory obligations to match the risks of a particular deep synthesis technology. While the law attempts to define different forms of deep synthesis technology, it takes a largely monolithic approach to regulating the different types of deepfakes. However, this section introduces two key differences between text fakes and audio or video fakes regulators should consider.
First, text fakes are harder to detect than other types of deepfakes. This is not a theoretical concern—text fakes are beginning to produce an acute plagiarism concern for educators. Deepfake videos are likely easier to discern, given our familiarity with biological cues and contextual information. Yet similar heuristics are often not available in the textual context; it can be difficult to detect whether a paragraph is written by an average undergraduate or an AI model.
Generative AI programs such as ChatGPT are “generative” precisely because they are trained to mimic human-produced content, but without ever replicating any single piece of text. They create something “new.” Because programs are trained by examining millions of existing text examples, generative AI’s “resulting text is [essentially] unique in comparison to what has been used in the training set.” GPT-3, for instance, is trained on a whopping 300 billion words, including books, articles and other texts on the internet. In the educational context, without a preexisting work that is the same as the AI-generated product, “the teacher will have to begrudgingly accept that the student wrote the essay as an original piece of work.” Institutions such as Sciences Po have already banned ChatGPT, and New York City’s education department has blocked access to the program on its devices and internet networks.
As a result of this difference in kind between text fakes and audio-visual fakes, regulation of text fakes should emphasize public awareness education by informing users at a young age to be skeptical of the veracity of the information generated by AI models. Given the public’s difficulty in discerning AI-generated text, labeling requirements for text fakes should be more robust than those for audio or video deepfakes, either through repetitive or prominent disclaimers advising the user of deep synthesis content.
Second, text fakes pose different types of privacy concerns than do audio or video deepfakes. Privacy violations are more obvious with audio and video deepfakes, as deepfakes in those scenarios are often intertwined with a specific individual. Text does not have that facet, as generative text does not stem from an unaltered original: there is nothing inherent in the content itself that shows the reader it came from a particular individual, unlike, for instance, a fake video of a public figure. Therefore, it is much harder for a single individual to claim they are being harmed by the program. However, allegations of generative AI programs making use of copyrighted content in their training data are already surfacing: whether copyright law will be held to address the use of copyright protected text in the training of or output from generative AI models remains untested in the courts.
C. Responsible Parties
Finally, given the numerous parties involved in creating and distributing synthesized content, the question remains: who should bear responsibility for compliance with applicable regulations? Should it be the creator of synthesized text such as the Hangzhou user that conducted the “experiment”? The service providers such as OpenAI? The platform that distributes the content, such as a social media company? As discussed above, the DEEPFAKES Accountability Act introduced in Congress did not specify the parties responsible for complying with its mandates. China’s Deep Synthesis Law chose to place the burden on “service providers,” an ambiguous term encompassing apps creating synthesized content as well as distribution platforms. State laws in the United States, on the other hand, generally provide private rights of action to victims of deepfakes, allowing plaintiffs to sue creators and distributors of synthesized content. The choice of whom to hold responsible has significant implications and should be carefully considered.
Some research suggests that the best method of mitigating content misinformation using generative text is to focus not on the content itself, but on distribution platforms. Given the speed at which generative AI can generate content, its proclivity for extremism, and the population’s vulnerability to misinformation, early detection and platform cooperation could be vital to preventing the spread of text fake misinformation. Distribution platforms may exacerbate disinformation campaigns, but by the same token they may be uniquely situated to ameliorate the threat presented by such campaigns.
Ahead of federal regulation, deep synthesis service providers could begin to self-regulate. Platforms are already looking for ways to improve the credibility of their generative text products. Microsoft, in incorporating generative AI into its Bing search engine, noted that its service will “be able to provide links to demonstrate where its answers are coming from, [a] feature that wasn’t part of ChatGPT.” Under the Deep Synthesis Law, China’s regulators took a cooperative rather than authoritative stance (as is more often the case with recent tech regulations in the country) with deep synthesis service providers. Article 5 of the law, for example, encourages service providers to establish industry standards, subject to regulatory oversight.
Similarly, U.S. policy-makers should evaluate the role of self-regulation regarding this technology. In the same way that FINRA regulates financial institutions subject to SEC rules, the federal government could establish a self-regulatory organization that oversees deep synthesis technology. The private organization would set standards for companies and be responsible for promoting and enforcing them. A federal regulatory body would oversee the organization, ensuring that standards are fair and adequate for service providers while protecting users of the technology.