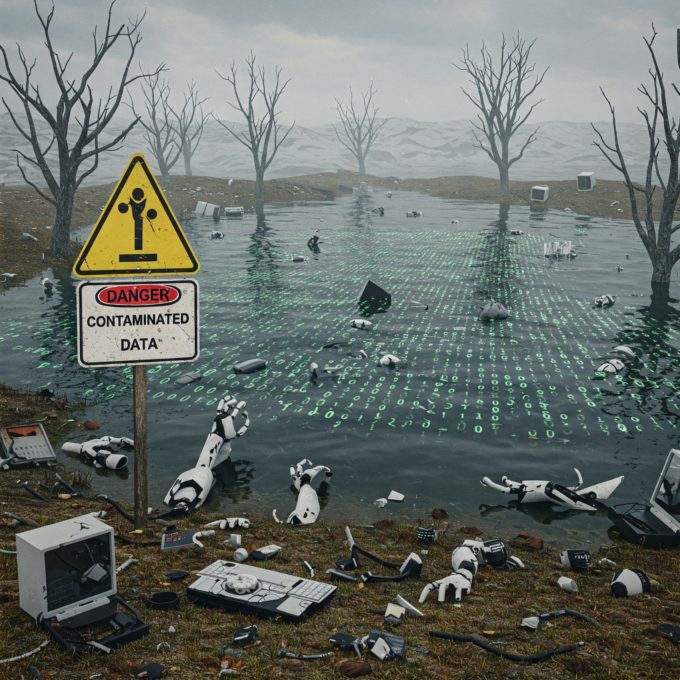
Model Collapse and the Right to Uncontaminated Human-Generated Data
By John Burden, Maurice Chiodo, Henning Grosse Ruse-Khan, Lisa Markschies, Dennis Müller, Seán Ó hÉigeartaigh, Rupprecht Podszun, and Herbert Zech - Edited by Kusuma Raditya and Pantho Sayed
The above image was AI-generated by Gemini.
John Burden is a Senior Research Associate on AI evaluation and AI safety, and Co-Director of the Kinds of Intelligence Programme, Leverhulme Centre for the Future of Intelligence, University of Cambridge. www.johnburden.co.uk.
Maurice Chiodo is a Research Associate in ethics in mathematically-powered technologies, Centre for the Study of Existential Risk, University of Cambridge. http://www.cser.ac.uk/team/maurice-chiodo.
Henning Grosse Ruse-Khan is a Professor of Law, University of Cambridge, Fellow at King's College, Cambridge. https://www.law.cam.ac.uk/people/academic/hm-grosse-ruse-khan/5328.
Lisa Markschies is a Research Assistant, Faculty of Law, Humboldt University of Berlin; associated researcher, Weizenbaum Institute, Berlin. https://www.weizenbaum-institut.de/en/portrait/p/lisa-markschies/.
Dennis Müller is a Research Associate, Institute of Mathematics Education, University of Cologne; Research Affiliate, Centre for the Study of Existential Risk, University of Cambridge. https://mathedidaktik.uni-koeln.de/mitarbeiterinnen/dennis-mueller.
Seán Ó hÉigeartaigh is Director of the AI: Futures and Responsibility Programme, working on the risks and governance of frontier AI, within the Centre for the Future of Intelligence, University of Cambridge. https://www.lcfi.ac.uk/people/sean-o-heigeartaigh.
Rupprecht Podszun is a Professor of Civil Law and Competition Law at Heinrich Heine University Düsseldorf. https://www.jura.hhu.de/en/lehrstuehle-und-institute/professuren-und-lehrstuehle-im-zivilrecht/chair-for-civil-law-german-and-european-competition-law.
Herbert Zech is Director at the Weizenbaum Institute, Berlin; Professor of Civil Law, Technology Law and IT Law at Humboldt University. https://www.weizenbaum-institut.de/en/portrait/p/herbert-zech/; https://www.rewi.hu-berlin.de/de/lf/ls/zch/cv
The following post is an abbreviated version of our findings from Legal Aspects of Access to Human-Generated Data and Other Essential Inputs for AI Training.
Are LLMs contaminating our data ecosystem?
The increasing use of Large Language Models (“LLMs”) and other generative Artificial Intelligence (“AI”) is now contaminating the online data environment with vast quantities of AI-generated “synthetic” content. This contamination potentially poses a significant problem for those wishing to train future AIs due to a phenomenon called model collapse, whereby AI trained on synthetic content produces increasingly useless or undesirable output — similar to re-photocopying a picture several times. Removing synthetic content from datasets can be difficult, so AI developers could require datasets which were collected prior to the proliferation of generative AI in 2022. This requirement can make AI development more difficult for future generations and might entrench existing players in their dominant positions over newcomers by virtue of the (uncontaminated) pre-2022 data they possess.
So which legal regimes might be useful to improve access to uncontaminated data and other essential inputs for AI training? How can existing approaches to AI regulation, data governance (particularly on access), European Union (“EU”) and domestic competition law, or gatekeepers for digital markets help? Is access to the “essential resource” of uncontaminated data — increasingly scarce and at significant risk of “extinction” — such an important public policy concern that the law should address before it’s too late? If so, how might such access be afforded in a fair and equitable manner, while minimizing the risks of harm from such access?
Background
Generative AI has recently exploded onto the world stage and is rapidly reshaping the way people work and create content. Such technology encompasses LLMs, which generate text, and Multi-Modal Models (“MMMs”), which generate combinations of text, images, audio, video, and other digital content. The foundation models underlying these generative AI technologies need to be trained on vast quantities of data — the better the data, the better the model. However, synthetic online content is now being inadvertently used to train upcoming generative AI models, and so this ecosystem is starting to feed back on itself, creating a problem which in antiquity was known as an Ouroboros: a snake which eats its own tail. In the 21st century, we have a new term for this: model collapse.
Model collapse
Model collapse, whereby LLMs and other generative AIs exhibit a tendency to collapse into producing gibberish nonsense or undesirable content if repeatedly re-trained on their own outputs, has become a concern among AI developers. Historically, training datasets were primarily sourced from internet scraping. Hence, a problem now emerges: the prevalence of generative AI means a growing proportion of internet content is influenced or produced by generative AIs themselves. Thus, future AI training — which will involve larger training datasets — will invariably include some of this “contaminated” data as companies continue scraping the Internet. Methods for detecting and removing AI-generated content are currently not very effective, so these training sets will remain contaminated. Soon, most of the Internet will become contaminated, as AI-generated text, pictures, and videos gradually appear everywhere. (Note that AI developers usually define data contamination as the unrelated problem of leakage of evaluation data into training datasets. While this is a significant problem for benchmarking AI performance, it doesn’t lead to issues of model collapse.)
So how does model collapse work in practice? It’s akin to repeatedly re-photocopying an image. AI training will usually under-sample (i.e., overlook) the low-probability features from its training data, and over-sample the high-probability ones. Here’s an illustration: If 1% of people wear red hats, 99% wear blue hats, and we randomly sample 20, there’s a good chance all 20 wear blue hats, so we might conclude that everyone wears blue hats and no one wears red hats. Yet, an AI trained on this sample might only generate text or images referring to people wearing blue hats, and never red hats. If the output of this AI starts to contaminate Internet data, the proportion of references to red hats will go down, so the next data collection might sample from a pool where 99.5% of people wear blue hats, and 0.5% wear red hats. An AI trained on that dataset is even more likely to conclude that everyone wears a blue hat. Eventually, as this process is repeated many times, all references to red hats will be totally lost. This “collapse” typically manifests as a continuous deterioration between iterations that may not even be noticed, rather than as an instantaneous failure of the AI.
Increasing data contamination creates a particular problem for newcomers to the generative AI market. Those who collected data from the internet after 2022 — when generative AIs became widely available — will suffer contamination issues; the later the data was collected, the more contaminated it will be. In addition, metadata such as date stamps cannot always be relied on to distinguish pre- vs post-2022 data, given how easily the metadata can be changed. Conversely, those who collected their data before 2022 have nearly guaranteed uncontaminated training sets. This phenomenon has occurred before in other contexts: for instance, with (pre-1945) low-background steel produced prior to the first nuclear explosions becoming essential for the manufacture of nuclear and medical sensors. Platforms that took vast user input before 2022 — including e-mail, messaging, spelling/grammar checkers, and image/video storage — have a particular advantage. They have unique access to vast non-synthetic pre-2022 data outside the public domain, so they are in an excellent position for effective future AI training, adding to the concerns of dependency vis-a-vis these companies. While work is being done to mitigate model collapse, these efforts might prove insufficient in the long term.
Of course, the impact on AI training is not the only negative effect from contaminating the data environment. The aggregate amount of indiscernible synthetic data potentially creates systemic damage: not just to AI training processes, but also in its potential to challenge the trust placed in online information and how it is exchanged. The more difficult it becomes to discern real content from synthetic, the higher the transaction costs. Checking information validity may become costly, and a social issue arises: if the rich are in a better position to check information accuracy, through their greater access to various tools and greater knowledge about the data ecosystem, they gain significant advantages in an information-dependent society. This isn’t only true for day-to-day information or economic decisions: debates in democracy and political decisions also rely on sound information. Personal views, decisions, social interactions, and friendships all rely on information, too, and if the infosphere is flooded with (untrustworthy) synthetic data, this may cause all sorts of severe problems. The lack of discernibly true information might propel society into chaos and endanger the social fabric keeping us together. While fair access to uncontaminated data will not be sufficient for resolving these broader concerns, it may well constitute a necessary policy.
AI training with human feedback
There is another essential ingredient for generative AI training: Reinforcement Learning from Human Feedback (“RLHF”). RLHF “refines” generative AI to align more with human values and preferences, whereby humans are tasked with evaluating and correcting a random sample of outputs of the AI base model after training. Think of the base model as the full set of learning the AI has already done, using a large training dataset and long expensive computation. RLHF is an additional “layer of manners” and “performance refinement” (or behavioral training) placed on top of that. It prevents outputs that humans would deem inappropriate or unacceptable (e.g., racism, sexism, offensive imagery, garbled text, etc.) and achieves outputs that meaningfully match the input request (i.e., actually answers the question posed). In other words, training on large datasets gives the AI its capabilities; RLHF teaches the AI what not to do (even though it can) and refines its capabilities.
At present, RLHF is mostly done with paid labor. But established generative AI providers with a large userbase, such as OpenAI, also rely on their users to carry out additional human feedback. They might ask users to give a “thumbs up/down” evaluation of an output, or present users with two responses and ask, “Which response do you prefer?” When millions of people are doing this regularly, the contribution can be significant — far exceeding what a paid workforce can accomplish. Additionally, human-generated data collected in this way may also be used in the training of later AI models as part of the standard training dataset.
Thus, established generative AI providers have another significant advantage over newcomers: they have access to a large userbase on which to carry out additional human feedback on their AI models, as well as from which to collect standard training data. This can create a “lock-out” effect, whereby newcomers can’t amass a sufficiently large userbase because they lack a product that can compete with the established players, and they can’t compete with the established players because they lack a sufficiently large userbase.
Established player advantage
To summarize, established generative AI providers:
- Are potentially contaminating the data environment through synthetic AI-generated content, directly in proportion to their commercial success or popularity;
- Have access to large, uncontaminated datasets that newcomers cannot access;
- Can access additional human-generated data in the form of large-scale human feedback from their vast userbase to improve their models;
- Potentially create a lock-out effect, maintaining superior AI tools over newcomers by utilizing their existing large userbase, and maintaining this base by creating superior AI tools.
These competitive advantages may be insurmountable by standard financial investment. Unlike other existing hurdles of data quantity and accessibility, compute resources, power costs, and human talent, it may become impossible, or at least prohibitively expensive, for newcomers to obtain additional uncontaminated datasets in the future, or to access large userbases from which to collect human feedback. Those who do possess such data or userbases might be unwilling to sell or share them. As well as inhibiting AI development, these characteristics create anti-competitive concerns: if newcomers cannot enter the market, power will rest with a few dominant incumbents.
So, from a legal standpoint, what can be done?
Regulating AI and its data environment
Laws and regulations often face well-known challenges when confronted with new technology, particularly in cross-border or global contexts. International coordinated responses are unlikely to emerge in the foreseeable future — as disunity at the Paris AI summit underscores. Even the EU — perhaps the swiftest actor to date in regulating the digital environment — doesn’t yet offer tailored solutions for contaminated data or the need for large-scale human feedback. However, a range of general approaches in the EU’s legal and regulatory portfolio exist. None currently seem fully fit for this purpose, and their concrete application to AI remains to be seen, but they indicate possible avenues for securing a functioning data and information environment for everyone in the future. While it remains uncertain just how badly contamination of the data environment will affect future AI tools, the general principle of precaution strongly militates for putting legislative and regulatory tools in place that can prevent harm before it materializes — in particular as the harm from contaminated data is likely to become ever more difficult to rectify in the future.
The EU’s specific legal regimes on AI and data might serve as an international example for good data governance rules helping to preserve the availability of uncontaminated datasets. This is especially true for access rights to uncontaminated data, obligations to mark AI-created data, and the requirement of data governance processes for AI systems. The impact on data accessibility in general, and AI dataset contamination in particular, of the EU Data Act, with its access and sharing rights to data generated by connected products (often referred to as IoT-Data) along with the establishment of envisioned data spaces, has yet to be seen. The same is true for marking AI-generated data under the EU AI Act. Nonetheless, such data governance rules are steps in the right direction. AI developers also have intrinsic incentives to use uncontaminated training data: an AI system that might collapse due to contaminated training data is a less desirable product and poses liability risks. For this reason, the existing data governance and quality obligations within Art. 10 of the AI Act are insufficient insofar as they lack direct obligations for data providers and are aimed at AI developers instead.
A legal framework governing data generation and allocation must ensure that enough uncontaminated data remains available. While the current rules could serve as a building block for this approach, they may still be insufficient. As discussed below, such legal frameworks might require the creation of a novel data space or “data trustee” to provide access to uncontaminated data for AI development.
Access to data under competition law
Competition law in Europe provides tools to obtain access to resources held by others when necessary to enable competition, which could include access to datasets in the AI context.
The idea to employ competition law here stems from data-related market entry barriers to AI markets. If access to uncontaminated data is vital for training new models, the flooding of the Internet and other principal data sources with AI-generated content becomes an insurmountable barrier to market entry for those without access to uncontaminated pre-2022 data. This, in turn, entrenches the competitive position of incumbents holding vast amounts of uncontaminated data, as they’re able to train their models better and compete in downstream markets where access to data is a key input. This may give incumbents another handle to expand their ecosystems — not just in markets directly related to AI products and services, but also in markets that increasingly rely on AI tools (e.g., healthcare services or manufacturing processes). That in turn may increase the market’s dependency on very few players, while impulses from new market entrants are cut off. Without competition, undertakings become less efficient and innovative. Market players no longer have the incentive to be better than others. In broad terms, this is the competition theory underpinning the tools discussed here.
Competition law interventions typically relate to three instances:
(1) There may be an infringement of the prohibition to use restrictive business practices in contractual agreements. When, for example, a data holding company has exclusivity clauses in its contracts or mandates its contractual partners not to make use of certain data, not to license a database to third parties, or not to collect data themselves, this may constitute an anti-competitive agreement. Under EU law, this would also be an infringement of Article 101 of the Treaty on the Functioning of the European Union (“TFEU”). At the same time, data holders might be forced by dominant AI firms to not license their data to others, or to license only on specific (less preferential) terms. Contracts between data holders and generative AI developers now exist.
(2) When a merger (e.g., the acquisition of a start-up by a dominant incumbent) leads to competitive concerns, competition agencies may prohibit the deal or take remedial action. One remedy could be the implementation of open access policies of datasets for third parties — to keep them in the game.
These two tools are relatively easy to employ but will not cover many situations where data access is a competitive issue.
(3) The access to data cases will usually fall into the category of abusive conduct under Article 102 TFEU in European competition law. A competition law access claim under this provision requires:
- a dominant position by the company in the relevant market;
- abusive conduct by hindering others from accessing the data; and
- the absence of a justification for such a refusal to access.
The competitive harm would usually arise from foreclosing a downstream (secondary) market by the dominant company. However, these requirements are very hard to meet: cases usually come with hard battles over evidence and take very long to resolve. Also, data holders may refer to copyright, trade secret, or privacy issues to withhold access.
Even if a competition agency or court in private enforcement finds an infringement of competition law, it’s not yet clear how to remediate the violation. Is it merely the granting of access to data through an API? Does the data have to be conferred in a certain way to a third party? Does the other party get access for federated learning only, rather than receive a copy of the data? How is the access remedy designed and enforced? It would be necessary for the design of the competition law remedy to specify what exactly the petitioner may request and under what conditions. Also, recompense would usually be required.
Interestingly, Germany introduced a provision (Sec. 20(1a)) in 2021, tailored to data access claims in the digital sphere. However, this provision hasn’t yet shown its effectiveness in practice, perhaps due to the peculiar position of petitioners or claimants. Many AI companies are in need of venture capital, and usually don’t design their business models premised on winning lengthy legal disputes against Big Tech. At best, they’ll try to integrate into the existing dominant ecosystems instead of challenging them.
While the outlook on utilizing competition law as an effective tool for tackling data access problems may be bleak, there remain possible avenues to pursue:
- Structural remedies are becoming more popular. U.S. courts are contemplating a divestiture of some Big Tech firms. A structural approach could be a breakthrough decision that may also deal with data. For instance, separating data holding units, splitting the data heap, or making the data accessible may form part of a structural intervention in the U.S. or the EU.
- The EU Digital Markets Act (“DMA”), which ensures contestability and fairness in markets dominated by digital gatekeepers, offers some interesting provisions. While it doesn’t directly address AI, it contains rules on data access. If the infrastructure for such data access is set up, this may solve some practical issues and inspire market-driven solutions for dealing with data. Also, the DMA can be amended relatively easily and could prove a powerful tool in the future.
Overall, there currently seems to be a lack of complainants, and a lack of tools fit to grant newcomers access to pre-2022 data.
Suggestions for a way forward
Beyond the existing legal and regulatory tools surveyed, one could consider a model compelling relevant actors to share their uncontaminated datasets and/or their userbase for human feedback, under a global or at least a multilateral regime of trusted access. Those asked to share could receive some form of equitable returns from those benefiting — e.g., akin to FRAND licensing in the context of standard essential patents, or benefit sharing for access to genetic resources under international environmental law frameworks such as the Convention on Biological Diversity (CBD), its Nagoya Protocol on Access and Benefit-Sharing for genetic resources and the multilateral system set up under the Food and Agriculture Organization (FAO) Treaty on Plant Genetic Resources for Food and Agriculture. Under such a model, uncontaminated datasets could be held by a trusted actor (e.g., an international organization) and a global fund could collect royalties each time the data is accessed for AI training. Access fees might depend on the importance of that training for the subsequent commercial use, with options for privileged (potentially free) uses for non-commercial research and other activities that are collectively agreed to be welfare-enhancing or worthy of support. Some of the royalties might be distributed to the main contributors to the datasets, with enough retained to ensure upkeep, accessibility of the data, and cover other operational costs.
Such an access regime could include:
- Obliging holders of large uncontaminated datasets to provide access to all with a demonstrable need, and in particular newcomers to the market. This could be through a combination of data sharing and/or federated learning, depending on the data sensitivity. This should be combined with a compensation regime based on reasonable costs, rather than monopolistic or oligopolistic pricing.
- Obligating actors providing generative AI services via highly subscribed online platforms to allow competitors fair access to provide their services, similar to (but fairer than) how Amazon Marketplace allows other traders to sell alongside Amazon itself. This would break network effects, give users the chance to ‘try out’ other generative AIs, and allow competitors to obtain their own human feedback. While competitors will likely have a worse AI model than the host, they can offer other incentives to users such as higher query limits. Gatekeeper regulation akin to the DMA should prevent self-preferencing of the dominant platform, and might even serve as a basis for accessing data and other essential inputs.
- Setting up a “data trustee” to store any pre-2022 data still available, and to collect as much additional data as possible now. 2024 data might be sufficiently uncontaminated for AI training, and future advances may enable removal of comparatively unsophisticated AI-generated content from then (but not later, as quality improves). The trustee should continue collecting any verifiably uncontaminated data that becomes available, setting everything aside for future generations to train AI on via shared or federated learning methods. (This is the digital version of bio-banks and artificial biosphere reserves.)
- Ensuring fair and equal access for all to any such database(s) set up, especially as they become more necessary for future AI training. Access points to the trustee should be set up across regions, and reasonable precautions adopted so that such data cannot be used for large scale harm — a concern that should be considered from the outset.
- Incentivizing a market-oriented, voluntary regime for access to uncontaminated data, perhaps through mandatory data sharing for gatekeepers, or based on a bottom-up approach where small- and medium-sized companies can market their data and thereby contribute to AI development. Public authorities might build (regulated) datasets (potentially with trustees) or — even better — competing datasets, determining the conditions for use and subsidizing the institution of such datasets. Expediently establishing such a back-up might well prove an essential incentive for the key actors to enter into agreements creating access to uncontaminated data.
Future challenges
There’s no simple solution to contaminated data. Our proposals above, which imply vast data hoarding, come with their own risks. Massive, centralized datasets pose significant privacy risks. While low-background steel sits under the ocean posing minimal risk to society, vast data centers could create significant future hazards. A massive, centralized storage facility can cause catastrophic harm if it is hacked, experiences a data leak, or is somehow compromised and deliberately contaminated. Further, trusted storage entities will need to remain trustworthy in the long term, as we may need such data for many decades. Thus, robust legal and technical tools are needed to scrutinize and protect the data-storing entity itself, which needs to have sufficient resources to be secure and (politically) independent in the long run.