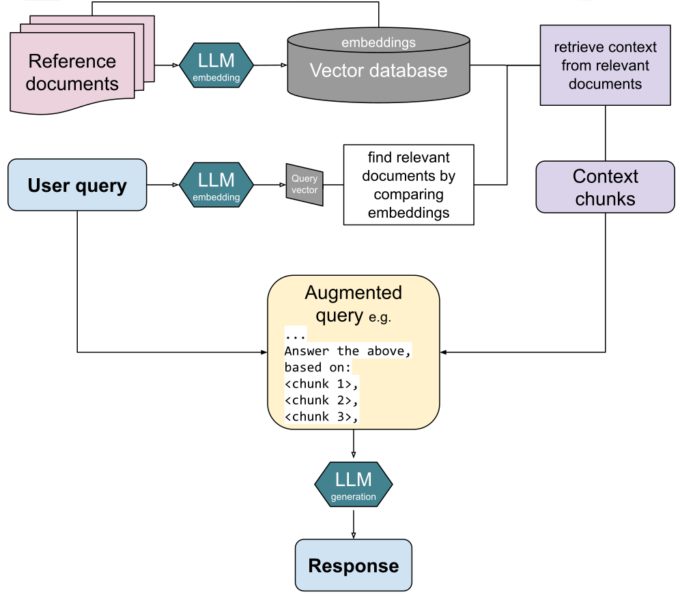
Retrieval-augmented generation (RAG): towards a promising LLM architecture for legal work?
By Peter Johnston - Edited by Kusuma Raditya and Pantho Sayed
The above image is sourced from Wikimedia Commons.
Generative artificial intelligence (AI)’s first encounter with the legal world was a catastrophe. After OpenAI released ChatGPT in Fall 2022 and the chatbot became the fastest adopted app in history, users all too quickly applied the chatbot to legal tasks for which it was poorly suited. Just six months after its launch, attorney Steven A. Schwartz filed a brief full of synthesized, hallucinated cases by ChatGPT. Schwartz later faced judicial sanctions for that filing. Chief Justice John Roberts even alluded to the incident in his 2023 report on the federal judiciary, calling the habit of submitting briefs citing non-existent cases “always a bad idea.”
Yet Schwartz’s highly publicized castigation and Chief Justice Roberts’s admonishment did not get through to all lawyers. Just this past February, in a products liability class action against Walmart for selling an exploding hoverboard, lawyers from the large plaintiffs’ firm Morgan & Morgan faced sanctions for submitting a federal court filing with hallucinated case citations in it. One Morgan & Morgan attorney had supplied an internal AI tool with prompts like “add to this Motion in Limine Federal Case law from Wyoming setting forth requirements for motions in limine” and even quite complex prompts like:
Add a paragraph to this motion in limine that evidence or commentary regarding an improperly discarded cigarette starting the fire must be precluded because there is no actual evidence of this, and that amounts to an impermissible stacking of inferences and pure speculation. Include case law from federal court in Wyoming to support exclusion of this type of evidence.
Academic work has substantiated the intuition that ChatGPT-like tools are not fit for legal work. A June 2024 paper found that even the best-performing LLM, GPT-4, hallucinated at least 49% of the time on the most basic case summary tasks presented. The authors noted LLMs’ overconfidence in their own outputs and their tendency to perform even worse when given a query containing incorrect information. Moreover, a randomized controlled trial in November 2024 studied how equipping law students with GPT-4 affected the quality and speed of their work on four simulated legal tasks typically handled by junior attorneys. The authors found a significant gain in speed, but no improvement in quality.
However, the recent emergence of retrieval-augmented generation (RAG) may portend a sea change in LLMs’ role in the legal world. RAG enhances the tremendous language capabilities of LLMs by incorporating external sources of truth, reducing hallucination rates.
Plain old non-RAG LLMs have so-called “parametric memory.” The LLM training process embeds knowledge contained within the training set into the model’s output parameters. In other words, the many billions of parameters in a modern LLM encode patterns, facts, and relationships from the vast quantity of data fed into it during training. This makes LLMs incredibly powerful when answering general queries, but parametric memory does not allow the LLM to reliably cite specific sources in its output or address deeper questions into specific areas of knowledge. These limitations contribute to LLMs’ tendency to hallucinate.
Enter RAG. First described in a 2020 paper by Facebook AI researchers, RAG enhances “conventional” LLMs with an external source of truth stored as a vector database. For example, the 2020 RAG paper used Wikipedia as its “non-parametric memory.” Rather than relying solely on the LLM’s parametric memory, the LLM with RAG first retrieves relevant documents from the vector database and then includes those documents in the LLM’s input context window in order to “ground” the response. Legal tasks are particularly well suited to RAG because of the availability of high-quality databases of statutes, cases, and regulations. And unlike conventional LLMs, whose expensive training process limits the frequency of introducing new knowledge, RAG vector databases can be updated frequently. One startup, Harvey, even offers a product that makes law firms’ proprietary databases available via RAG systems.
A recent randomized-controlled trial by Prof. Daniel Schwarcz and co-authors, published last month, examined the efficacy of equipping law students with a RAG-powered legal LLM tool, “Vincent AI,” on six representative legal tasks. This approach largely mirrored the approach used in the November 2024 paper examining GPT-4’s effects on law students. Unlike with GPT-4, where the researchers found no improvement in work product quality, with the RAG-powered tool, the researchers found statistically significant gains in four of the six tasks, including “enhanced clarity, organization, and professionalism of submitted work.” While not suited for all tasks—the RAG tool was best suited “when the assignment presented a narrower issue clearly outlined in the prompt—Vincent AI did not suffer from the comparison tool’s (OpenAI’s o1-preview) occasional tendency to hallucinate “entirely fabricated” cases. The authors concluded by noting that the RAG tool was capable of “reduc[ing] hallucinations in human legal work to levels comparable to those found in work completed without AI assistance.”
The emergence of RAG-enabled LLMs offers a critical opportunity to re-evaluate AI’s transformative potential for legal work. Repeated high-profile, embarrassing hallucinations fatally discredited LLMs’ applicability to legal work, where facts and authority are of paramount importance. RAG tools, with their reference to external sources of truth and the ability to provide citations, challenge this skepticism. Prof. Schwarcz’s sequential randomized-controlled studies (on two different teams) provide particularly strong evidence that RAG-enabled LLMs offer more than prior general-purpose LLMs like GPT-4.
With a viable partial solution to the hallucination problem now available, it will be exciting to observe how RAG-enabled LLMs handle legal tasks in the real world.