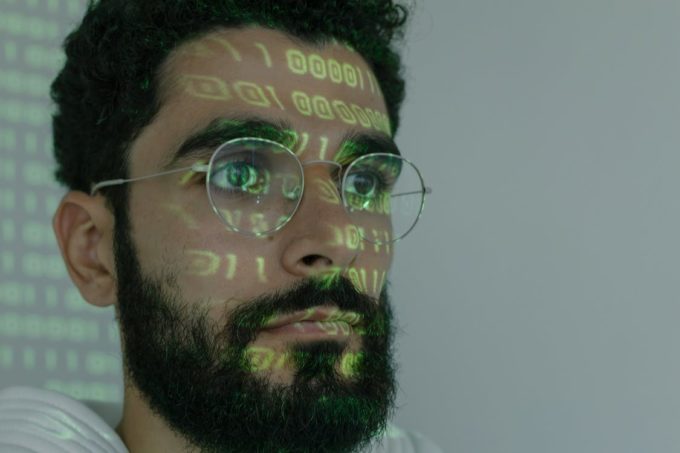
Why Racial Bias is Prevalent in Facial Recognition Technology
By Beth Findley - Edited by Mariah Bellamoroso
In 2019, the National Institute of Standards and Technology (“NIST”) published a report analyzing the performance, across races, of 189 facial recognition algorithms submitted by 99 developers, including Microsoft, Intel, Idemia, and other major tech and surveillance companies. Many of these algorithms were found to be between 10 and 100 times more likely to misidentify a Black or East Asian face than a white face. In some cases, American Indian faces were the most frequently misidentified. Most algorithms were substantially less likely to correctly identify a Black woman than a member of any other demographic. To understand the reasons for these disparities, it helps to understand the basics of how facial recognition technology works.
Modern facial recognition technology relies on a layered structure of algorithms called a neural network to programmatically identify features that the computer considers relevant to distinguishing faces. Humans do not decide—and rarely understand—the features a neural network uses to identify images. The neural network “learns” these features from the images used to “train” the algorithm. Older facial recognition technology uses more traditional machine learning techniques that still “learn” from training images but require the training images to be labeled with human-specified features. Rather than determining which features are relevant itself, the computer uses the human-specified features to distinguish faces.
Race influences the development and performance of facial recognition technology in three distinct ways. The cardinal factor driving racially disparate results is non-diverse training images: human bias and data availability affect the racial distribution of faces used to train the algorithm, usually with lighter skin tones predominating. Other factors include human selection of facial features in older algorithms and image quality issues that disproportionately affect darker skin tones. Collectively, these problems lead to facial recognition technology that performs unevenly across races—typically worse for darker skin tones.
Racial bias is most prevalent in the selection of images used to train the algorithm. In general, result accuracy is proportional to data quality, and a racially unbiased technology would require equal racial representation within the dataset. This is rarely accomplished because many publicly available image datasets are non-diverse; Labeled Faces in the Wild, a popular open source facial image dataset, is 83.5% white. The NIST-constructed IJB-A dataset was specifically created for geographical diversity; nonetheless, 79.6% of its images are of faces with lighter skin tones. For facial recognition technology to be trained on appropriately diverse images, an engineer must think to consider racial balance, evaluate the diversity of available datasets, and, when the available datasets are insufficient, prioritize racial diversity over the costs of constructing a new dataset. Failure to follow this process can result in an unevenly trained algorithm.
Race can also be relevant in older algorithms that rely on human selection to determine which facial features should be analyzed. There are thousands of qualities one could possibly consider, such as eye shape, nose-to-chin distance, or eyebrow color. Which features an engineer chooses may be influenced by their own race given psychological research on the “other-race effect” and racial influence on facial feature recognition. This effect, in conjunction with different racial compositions of training image sets, may help explain a 2011 NIST study finding that facial recognition algorithms developed in China, Japan, and South Korea recognized Asian faces more easily than Caucasian faces, while the reverse was true for algorithms developed in the United States, France, and Germany.
Finally, image quality was identified by the 2019 NIST study as a significant source of facial recognition error, and racial disparities lurk behind that problem as well. Quality problems affect not only training images, but also the actual images that facial recognition technology attempts to identify in the real world. Some image quality issues are generally nondiscriminatory; blurriness and differences in pose or camera angle can impair facial matching accuracy evenly across races. However, problems like underexposure will reduce image quality more dramatically for darker skin tones. Historically, camera technology has been calibrated for light skin only, and many image quality issues persist only for those with darker skin. Thus, poorer image quality is another factor yielding disproportionately inaccurate facial recognition results for people with darker skin. Activists have repeatedly demonstrated racial bias in facial recognition. UCLA backed down from a plan to use facial recognition on its campus after the digital rights nonprofit Fight for the Future tested its algorithm on 400 pictures of UCLA staff, faculty, and students. The test revealed 58 false matches with images from a mugshot database, mostly for people of color. The ACLU ran a similar test using pictures of members of Congress: 28 members, again mostly people of color, were wrongly identified in the mugshot database. These and other small-scale tests of facial recognition performance across races comport with the 2019 NIST study conclusion that racial bias is consistent throughout many implementations. Any policy concerning the use of facial recognition must grapple with the racial disparities inescapably embedded within the technology itself.